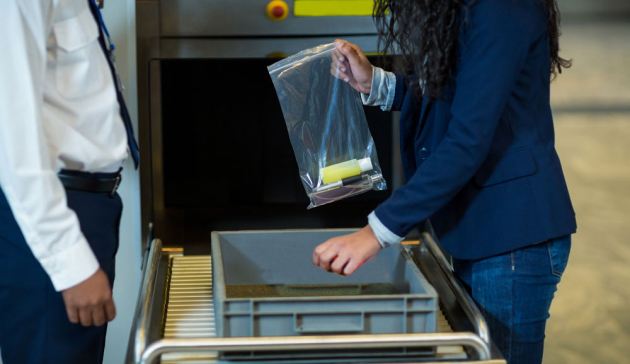
Safeguarding X-ray Scanning Systems in Border Security
Rapid advancements in the realm of artificial intelligence (AI) and machine learning (ML) have ushered in unprecedented capabilities, revolutionizing industries from healthcare to transportation and reshaping approaches to complex challenges like anomaly detection in non-intrusive inspections. Yet with great technological progress comes the real threat of adversarial attacks, which compromise the reliability and effectiveness of these AI models.
Imagine a scenario where an AI-powered system creates synthetic data for computer vision at national borders. It creates an emulated X-ray sensor that can produce synthetic X-ray scan images similar to real X-ray scan images, and virtual 3D replicas of vehicles and narcotics containers. This set of images can be used to train the system to detect anomalies for application of global transport systems. For example, the system can be used in customs and border protection to identify narcotics and other contrabands in conveyances and cargo. However sophisticated this system, it is vulnerable if malicious actors exploit its weaknesses through adversarial attacks.
Understanding adversarial attacks
Adversarial attacks are deliberate manipulations of AI models through subtle modifications to input data. These modifications are often imperceptible to human eyes but can cause AI algorithms to misclassify or fail in their intended tasks. In the context of X-ray scan emulation and model classification, an adversarial attack could potentially introduce deceptive elements into images. For instance, altering a few pixels in an X-ray image might trick the AI into missing or misidentifying illicit substances, thereby compromising security protocols.
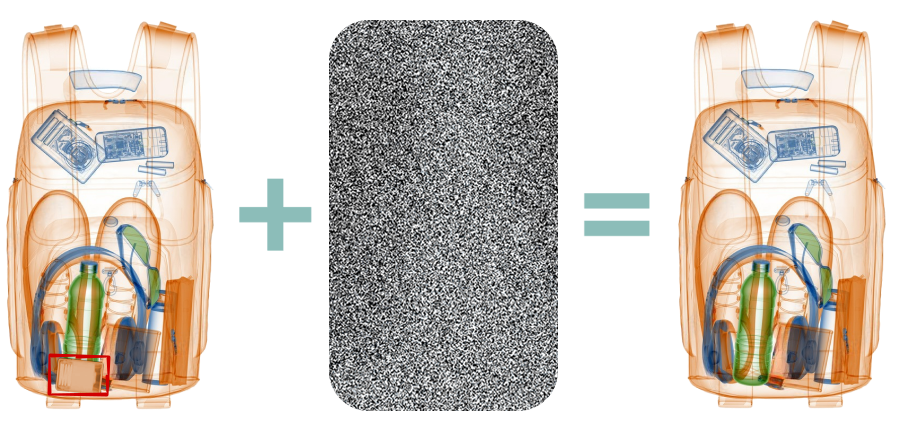
The stakes: Why AI model security matters
The implications of compromised AI models in security applications can be profound. Inaccurate or manipulated anomaly detection can lead to serious consequences; in the case of customs and border security, this could mean undetected smuggling of narcotics or other illegal items, posing risks to both public safety and national security. Here, safeguarding AI models from adversarial attacks is not just a matter of technological integrity but also a crucial component of maintaining public order and staying compliant with regulatory standards.
Challenges in securing AI models – and how Styrk offers protection
Vulnerability to perturbations:
AI models are susceptible to small, carefully crafted perturbations in input data that can cause significant changes in output predictions. Styrk can identify vulnerabilities of the AI model and propose mitigation mechanisms to safeguard from such perturbations.
Lack of robustness:
If not carefully monitored, measured, and mitigated, AI models typically lack robustness against adversarial examples, as they are often trained on clean, well-behaved data that does not adequately represent the complexity and variability of real-world scenarios. Styrk can help you identify the kind of adversarial attacks your model might be susceptible to and suggest relevant mitigation mechanisms.
Complexity of attacks:
Adversarial attacks can take various forms such as: evasion attacks; where inputs are manipulated to evade detection, poisoning attacks; where training data is compromised, or any other such attack, necessitating comprehensive defense strategies. Most defenses in the market are designed to protect against specific types of adversarial attacks. When new attack techniques are developed, defenses can become ineffective, leaving models vulnerable to unseen attack methods. In contrast, Styrk’s Armor presents a comprehensive suite that scans the model to identify vulnerabilities in the model. It also offers a single proprietary, patent pending defense for adversarial attacks on traditional AI/ML models that covers a wide range of attacks.
Resource constraints:
Organizations may face limitations in terms of computational resources, time, and expertise required to implement robust defenses against a wide range of adversarial threats in their AI models. Especially in such scenarios, Styrk’s Armor offers an auto-scalable vulnerability scanning tool that can be used to identify potential vulnerabilities in the model and its proprietary defense mechanism proposes the best mitigation strategy that is practical across a wide range of attacks.