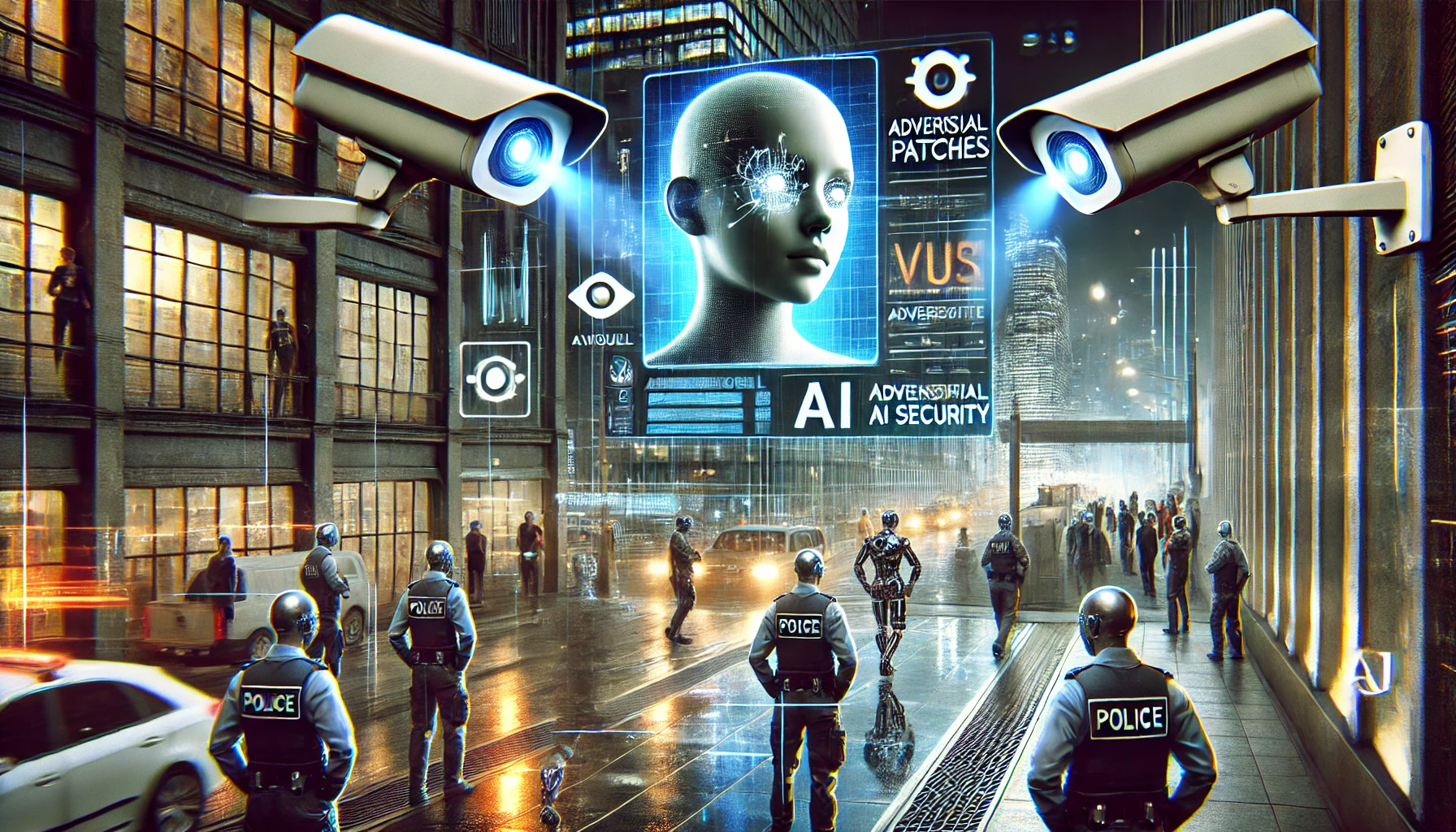
The Growing Importance of Robustness in Visual Detection Systems
In 2025, artificial intelligence (AI) is at the heart of mission-critical systems across industries, from healthcare to public safety. Visual AI technologies, such as those used for gun detection in public spaces, have become indispensable tools for enhancing safety and security. However, as these AI-powered systems become more prevalent, it becomes ever critical to ensure their resilience to model vulnerabilities, both adversarial and incidental.
The stakes are incredibly high when it comes to AI systems designed to protect human life. A compromised visual AI system could lead to catastrophic consequences, from failing to detect genuine threats to generating false alarms that cause panic and disruption. As these technologies continue to evolve and integrate more deeply into our security infrastructure, ensuring their reliability and tolerance against intentional and unintentional exploits becomes paramount.
Adversarial attacks, model vulnerabilities, and compliance challenges are increasingly threatening the reliability and security of AI systems. A recent study revealed that 77% of businesses experienced a breach of their AI systems in the past year, posing significant risks to organizations. This highlights the urgent need to prioritize AI security as part of the development and operational processes.
As we stand on the precipice of an AI-driven future, we must ask ourselves: Are we prepared to defend the very systems we’ve entrusted with our safety? For security professionals and developers committed to safeguarding AI adoption, consider this question as we delve into the complex landscape of AI security, exploring the risks and consequences from adversarial threats and vulnerabilities to visual AI detection.
What Are Adversarial Attacks in Visual AI?
Adversarial attacks are deliberate manipulations of input data designed to deceive AI models. In the context of visual AI, these attacks often involve subtle changes to images or physical objects that are imperceptible to humans but can cause AI systems to misclassify or fail to detect objects.
Examples of Adversarial Attacks in Visual AI:
- Medical Imaging Misclassification: Slight pixel-level changes to CT scans have been shown to trick AI models into misdiagnosing benign conditions as malignant tumors, or vice versa, with potentially life-threatening consequences (Neuroscience News).
- Autonomous Vehicle Misclassification: Adversarial attacks can manipulate the visual perception systems of autonomous vehicles by introducing subtle perturbations to road signs. For instance, attackers can apply small stickers or graffiti to a stop sign, causing the vehicle’s AI to misinterpret it as a yield sign or another instruction. This misclassification can lead to dangerous driving behaviors, posing significant safety risks (Springer Nature).
- Facial Recognition System Evasion: Adversaries can employ techniques to evade facial recognition systems used in security contexts. By wearing specially crafted accessories, such as glasses with adversarial patterns, individuals can fool AI models into misidentifying them as different persons or failing to recognize them entirely. This vulnerability can be exploited to bypass security measures in sensitive areas (Center for Emerging Technology and Security).
These attacks exploit the inherent vulnerabilities in AI models, which rely on patterns in data that can be subtly altered to produce incorrect outputs.
Why Visual AI Systems Are Particularly Vulnerable
Visual AI systems, such as those used in gun detection, face unique vulnerabilities due to the complex and dynamic nature of their operating environment. These challenges include:
- Complexity of Visual Data: Images and videos are rich with information, making them susceptible to exploitation. Attackers can manipulate small details or patterns within this data to deceive AI systems, leading to misclassification or failure to detect threats.
- Dependence on Training Data: Visual AI models rely heavily on the quality and diversity of their training datasets. Limited or biased data can hinder their ability to generalize to real-world scenarios, leaving them vulnerable to novel or unexpected inputs.
- Real-World Variability: Unlike digital-only systems, visual AI must navigate unpredictable physical conditions such as changes in lighting, angles, occlusions, and motion. These variables can degrade performance and are easily exploited by adversaries.
- Emerging Technologies: The accessibility of tools like 3D printers and custom fabrication methods enables the creation of objects with unconventional designs, materials, or geometries that visual AI systems may not recognize. For example, 3D printed firearms and other non-standard objects can evade detection if they lack traditional visual cues.
- Intentional Evasion Techniques: Civilians now have access to adversarial tools such as printed patches, specialized clothing, or DIY camouflage designed specifically to confuse AI models. These techniques exploit weaknesses in visual recognition algorithms, making it harder for systems to reliably identify people or objects.
- Dynamic Threat Landscape: The rapid evolution of technologies like augmented reality (AR) and programmable materials introduces ever-changing visual inputs that challenge AI systems to keep pace. This constant innovation increases the likelihood of blind spots in detection capabilities.
By understanding these vulnerabilities, developers can work toward creating more robust visual AI systems that are better equipped to handle the complexities of real-world environments and emerging threats.
Statistic: A study by OpenAI found that adversarial examples can reduce the accuracy of image classification models by up to 90%, even when the changes to the input data are imperceptible to humans (OpenAI Blog).
The Consequences of Adversarial Attacks on Visual AI
For mission-critical systems like gun detection technology, the consequences of adversarial attacks can be severe:
- False Positives: Misclassifying harmless objects as weapons could lead to unnecessary panic, operational disruptions, and loss of trust in the system.
- Example: In 2022, a school security system in the U.S. triggered a false alarm after misidentifying a student’s umbrella as a firearm, leading to a lockdown and significant public backlash (The Verge).
- False Negatives: Failing to detect a weapon due to adversarial manipulation could have catastrophic consequences, including loss of life.
- Example: Researchers at Carnegie Mellon University demonstrated how adversarial patches could be used to make firearms invisible to AI-powered detection systems.
- Reputational Damage: A single high-profile failure can erode public confidence in AI-powered safety systems.
- Regulatory Non-Compliance: Emerging AI regulations in 2025 require organizations to demonstrate robust risk management practices. Failing to address vulnerabilities could result in fines or restrictions.
How to Protect Visual AI Systems from Adversarial Attacks
To safeguard mission-critical visual AI systems, organizations must adopt a proactive, security-first approach. Here are some best practices:
- Incorporate AISecOps into Development
- AISecOps (AI Security Operations) integrates security into every stage of the AI lifecycle, from model design to deployment. This ensures that vulnerabilities are identified and mitigated early.
- Adopt Security-by-Design Practices
- Build models with security in mind, incorporating techniques like adversarial training, which exposes models to adversarial examples during training to improve their robustness.
- Conduct Regular Risk Assessments
- Perform regular vulnerability scans and penetration testing on AI models to identify weaknesses.
- Statistic: According to Deloitte, only 39% of organizations currently conduct regular AI risk assessments, leaving many systems exposed to potential threats.
- Monitor for Model Drift
- Continuously monitor model performance to detect and address drift, which occurs when a model’s accuracy degrades over time due to changes in input data.
- Collaborate Across Teams
- Bridge the gap between data scientists, AI engineers, and cybersecurity teams to ensure a holistic approach to AI security.
Case Study: Adversarial Attacks in Security Systems
In a recent study, researchers demonstrated how adversarial attacks could compromise AI-powered surveillance systems. By introducing small physical changes, such as wearing adversarial patches on clothing, attackers were able to evade detection entirely.
For companies creating innovative visual detection technologies, this underscores the importance of hardening AI models against adversarial threats to ensure their systems remain reliable in real-world scenarios.
Building Trust in Visual AI Systems
At Styrk AI, we specialize in helping organizations protect their AI/ML models from vulnerabilities and adversarial threats. Our solutions include:
- AI Risk Assessment & Management: Identify and mitigate vulnerabilities in your AI models and applications.
- Model Hardening: Strengthen your models against adversarial attacks and other threats.
- AISecOps Adoption: Incorporate security-by-design practices into your AI development process.
As AI continues to play a critical role in public safety, ensuring the security and reliability of visual detection systems is more important than ever. Adversarial attacks and other vulnerabilities pose significant risks, but with the right strategies and tools, organizations can protect their models and maintain trust in their technologies.
By partnering with Styrk AI, you can ensure that your visual AI systems are robust, secure, and compliant with emerging regulations. If you’re interested in learning more about how to secure your visual AI systems, contact us for a free consultation or check out our AI Security Checklist to get started.