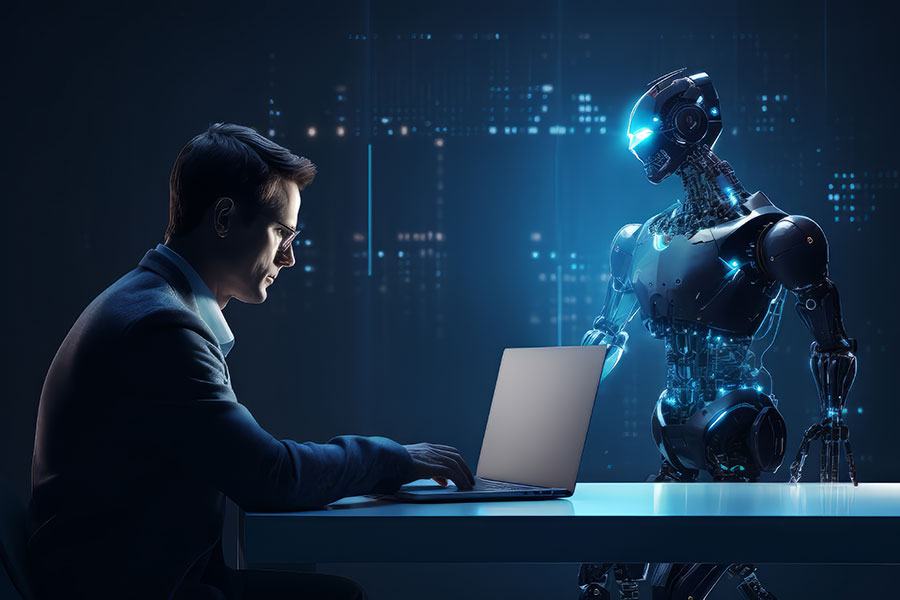
Explainability and Bias in AI: A Security Risk?
In the rapidly evolving landscape of artificial intelligence, the concepts of explainability and bias are at the forefront of discussions about security and trust. As AI systems and large language models (LLMs) are increasingly integrated into various sectors, from healthcare to finance, ensuring these systems are both understandable and unbiased is crucial. But why are explainability and bias themselves considered security risks, and what can be done to mitigate these risks?
The Importance of Explainability in AI
Explainability refers to an AI model’s ability to understand and interpret the decisions made by its systems. For users and stakeholders to trust AI, they need to know how decisions are reached. In critical applications such as medical diagnosis or loan approvals, the inability to explain AI decisions can lead to mistrust and even harmful outcomes.
Example: Healthcare
Imagine an AI system used to diagnose diseases. If the system identifies a condition but cannot explain how it arrived at that conclusion, doctors may find it difficult to trust the diagnosis. Worse, if the AI is wrong, patients might receive inappropriate treatments, leading to severe consequences. Transparent AI models that provide insights into their decision-making process can help medical professionals make better-informed decisions, thus enhancing trust and safety.
The Challenge of Bias in AI
Bias in AI occurs when a model produces prejudiced outcomes due to flawed data or algorithms. Bias can manifest in various forms, such as racial, gender, or socioeconomic biases, and can significantly impact the fairness and equity of AI applications.
Example: Hiring Practices
Consider an AI system used for hiring employees. If the training data predominantly includes resumes from a specific demographic, the AI might learn to favor candidates from that group, perpetuating existing inequalities. Such bias not only undermines the fairness of the hiring process but also exposes companies to legal risks and reputational damage.
Explainability and Bias as Security Risks
Both explainability and bias directly impact the security and trustworthiness of AI systems. Unexplainable AI decisions can be manipulated or misinterpreted, leading to security vulnerabilities. For instance, if an AI system’s behavior cannot be understood, malicious actors might exploit this opacity to manipulate outcomes without detection.
Bias, on the other hand, can erode the foundational trust in AI systems. Biased outcomes can lead to discriminatory practices, resulting in social and ethical issues that compromise the security and integrity of AI applications.
Mitigating Risks with Explainability and Bias Management
To address these challenges, it is essential to implement robust mechanisms that enhance the explainability of AI models and actively manage and mitigate bias.
Approaches to Enhance Explainability:
Model Transparency:
Using interpretable models or providing explanations for complex models helps users understand AI decisions.
Post-Hoc Explanations:
Techniques such as LIME (Local Interpretable Model-agnostic Explanations) and SHAP (Shapley Additive Explanations) can be used to explain the outputs of black-box models.
Human-AI Collaboration:
Encouraging collaboration between AI systems and human experts ensures that AI decisions are validated and understood.
Strategies to Mitigate Bias:
Diverse Training Data:
Ensuring that the training data is representative of all relevant demographics helps reduce bias.
Bias Detection Tools:
Using tools to regularly check for bias in AI models can help identify and correct prejudiced outcomes.
Continuous Monitoring:
Implementing continuous monitoring systems to track AI decisions and outcomes ensures ongoing fairness and equity.
Introducing Styrk’s Trust Solution
At Styrk AI, we recognize the critical importance of explainability and bias management in AI systems. Styrk’s Trust is designed to measure, monitor, and mitigate bias in AI models and LLMs. With comprehensive and configurable scans, our solution assesses the results using industry-standard metrics, ensuring that your AI systems remain fair, transparent, and trustworthy.
By leveraging Styrk’s Solution, organizations can enhance the security, trustworthiness, and ethical standing of their AI applications, ultimately driving better outcomes and fostering greater trust among users and stakeholders.
Managing risk proactively
Explainability and bias in AI are not just technical challenges; they are fundamental security risks that require proactive management. By adopting comprehensive solutions, organizations can address these risks head-on, ensuring that their AI systems are both fair and transparent, thereby safeguarding their integrity and trustworthiness in an increasingly AI-driven world.