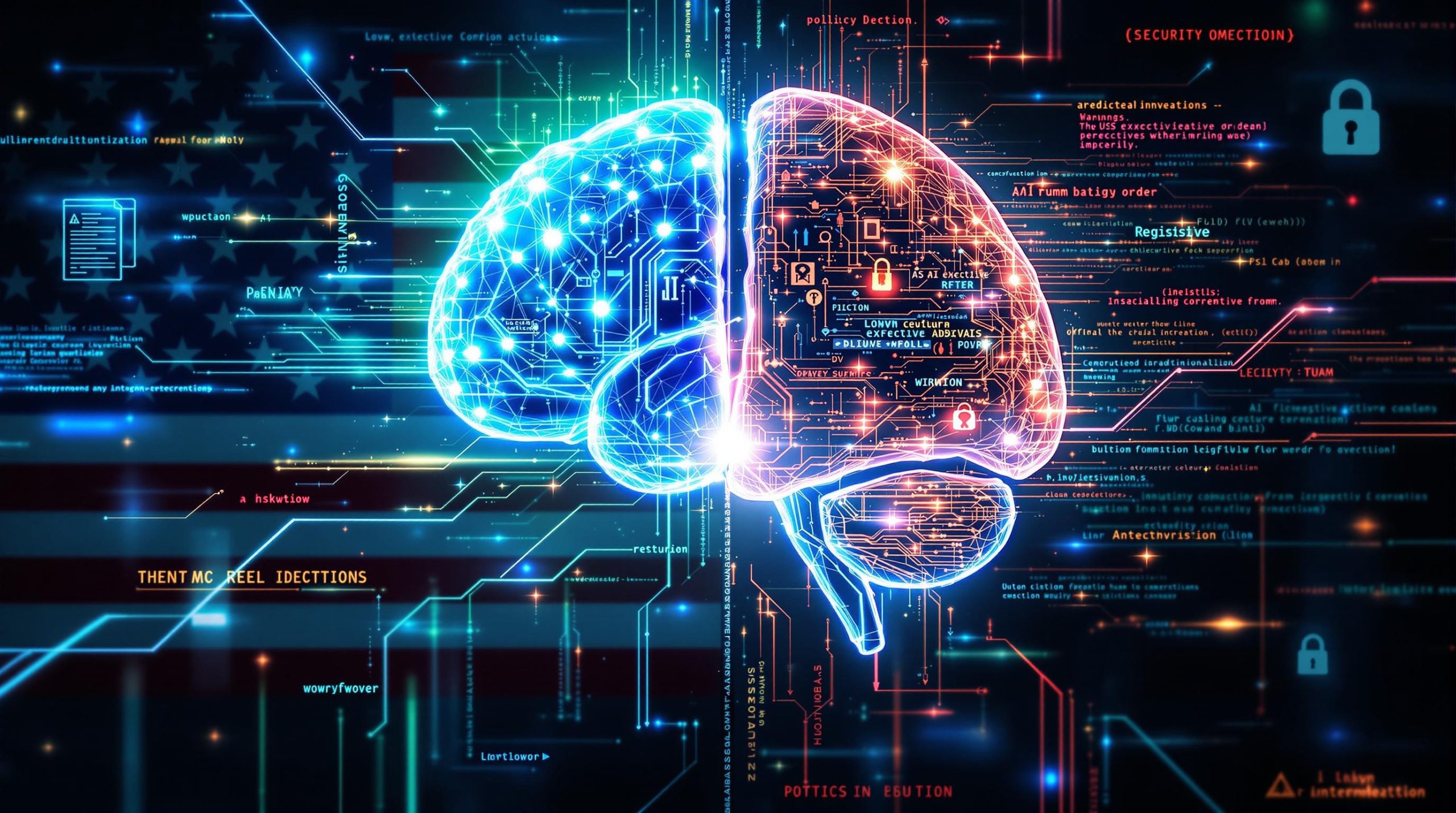
The confluence of President Trump’s executive order on AI and the public release of DeepSeek-R1 has created a complex and dynamic landscape in the AI market, one where rapid innovation runs parallel with escalating security concerns. Let’s break down the key elements and their implications:
Trump’s Executive Order: A Deregulatory Approach to AI Dominance
The core aim of Trump’s “Removing Barriers to American Leadership in Artificial Intelligence” executive order is to foster American AI dominance by rolling back regulations perceived as hindering innovation. While proponents argue this will unleash the American AI industry, critics express concern over potential safety and ethical implications. The revocation of the Biden administration’s AI executive order, which emphasized safety, security, and trustworthiness, shifts the responsibility of ensuring responsible AI development more squarely onto individual companies.
DeepSeek-R1: Democratization and Disruption
DeepSeek-R1’s release marks a significant step towards the democratization of AI, making powerful reasoning capabilities more accessible. However, this accessibility also presents new security challenges. The open-source nature of the model, while fostering innovation, also potentially allows malicious actors to identify and exploit vulnerabilities. Furthermore, concerns have been raised about data privacy and the potential misuse of the model.
Impact on the AI Market: A Crucible of Innovation and Risk
The convergence of Trump’s executive order and the DeepSeek-R1 release has thrown the AI market into a crucible of both immense opportunity and heightened risk. The executive order’s deregulatory approach, while potentially accelerating development by reducing burdens, places the onus of responsible development squarely on companies. Simultaneously, DeepSeek-R1’s open-source nature democratizes access to powerful AI capabilities, fostering a surge of innovation. This combination creates a dynamic market where speed and accessibility are paramount.
This new paradigm presents a complex challenge. While open source can drive rapid progress and wider adoption, as seen with projects like PyTorch, it also raises concerns about safety and security. The potential for misuse by malicious actors and the complexities of regulating open-source models are key issues policymakers are grappling with. The market’s reaction, including stock fluctuations and increased investment in AI security, reflects this tension between innovation and risk.
Furthermore, the interplay between open-source and closed-source models is being redefined. Companies like Mistral are betting on open source as a competitive advantage, while others like OpenAI maintain a closed approach. This dynamic raises questions about the long-term viability of different business models and the potential for a fragmented AI ecosystem. The debate around open versus closed AI is not merely a technical one; it has significant implications for market competition, innovation, and ultimately, the future direction of the AI industry. The EU’s AI Act, with its complex regulations regarding open-source AI, further complicates this landscape.
This confluence of deregulation, open-source advancements, and evolving regulatory landscapes creates a unique moment in the AI market. It’s a period of rapid advancement and democratization, but also one that demands careful consideration of the associated risks and the development of robust security measures to ensure responsible and beneficial AI development.
Prioritizing AI Security: The Unavoidable Truth
The current landscape presents a stark juxtaposition: the immense potential of AI versus the escalating risks. As AI becomes more integrated into critical systems, the potential consequences of security breaches and malicious attacks become increasingly severe. This necessitates a proactive and comprehensive approach to AI security.
Organizations must prioritize AI security across the entire development lifecycle. This includes:
- Robust data privacy measures: Protecting the data used to train and operate AI models is crucial.
- Rigorous testing and validation: Adversarial testing and red teaming can help identify and mitigate vulnerabilities.
- Transparency and explainability: Understanding how AI models work is essential for identifying and addressing potential biases and security risks.
- Investment in AI security solutions: Companies specializing in AI security offer tools and expertise to help organizations protect their AI systems.
The convergence of these events serves as a wake-up call. While the potential benefits of AI are immense, we must not ignore the accompanying risks. By prioritizing AI security, we can harness the transformative power of AI while mitigating its potential dangers, paving the way for a secure and trustworthy AI-powered future.