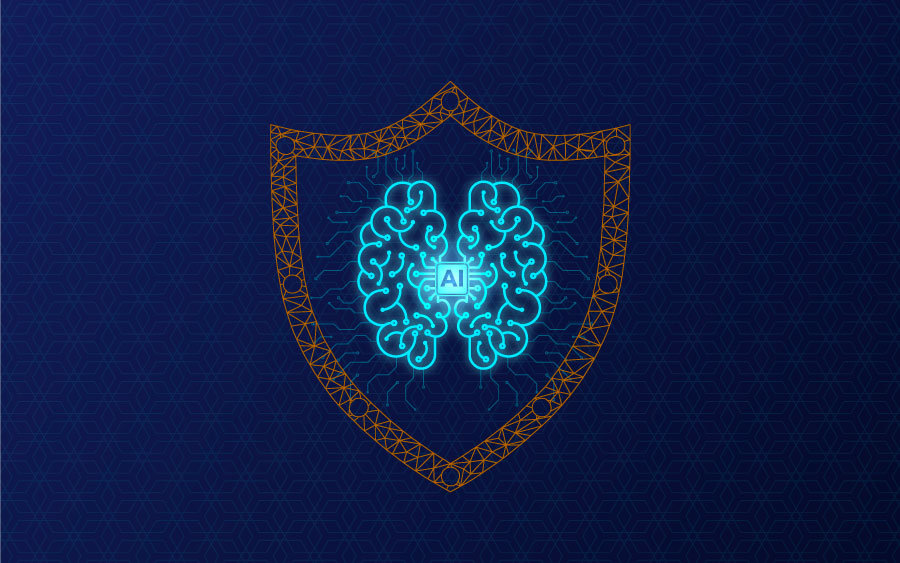
Privacy-Preserving Methods in AI: Protecting Data While Training Models
AI models are only as good as the data they are trained on. However, training models on real-world data often requires access to personally identifiable information (PII). Unchecked, AI systems can inadvertently expose or misuse sensitive data. With increased scrutiny and tightened compliance requirements due to regulations like the EU AI Act and GDPR, protecting this data is paramount.
Styrk provides tools and frameworks to help enterprises protect sensitive data while training AI models, and can help your organization employ key privacy-preserving techniques:
1 – Federated learning
Federated learning is a decentralized approach where multiple devices or servers collaborate to train a model without exchanging raw data. Instead, models are trained locally on individual devices, and only the trained model parameters are shared. This technique is particularly useful in sectors like healthcare, where patient data must remain private and secure.
2 – Differential privacy
Differential privacy adds mathematical noise to data or results during AI training to obscure individual data points, while still allowing for the generation of meaningful insights. This approach is highly effective in preventing the identification of individuals within datasets.
3 – Homomorphic encryption
Homomorphic encryption allows AI models to perform computations on encrypted data without needing to decrypt it. This ensures that even during processing, sensitive data remains secure and unreadable.
4 – Data anonymization
Data anonymization is the process of removing or masking personally identifiable information from datasets before they are used in AI training. By anonymizing data, organizations can still train AI models without violating privacy regulations.
5 – Synthetic data generation
Synthetic data involves creating artificial datasets that closely mimic real data but contain no real personal information. This method allows organizations to train AI models on realistic datasets without risking privacy breaches.
How Styrk can help you stay compliant and secure
Our advanced data masking and anonymization tools help prevent re-identification of anonymized datasets, and assist in generating high-quality synthetic data that retains the essential properties of real datasets while ensuring privacy protection. With comprehensive privacy monitoring and adversarial attack protection, we help enterprises comply with regulations, while securing their AI systems against evolving threats. Don’t let privacy concerns hold you back from AI innovation. Contact us today to learn how Styrk can help secure your AI models while safeguarding your data.