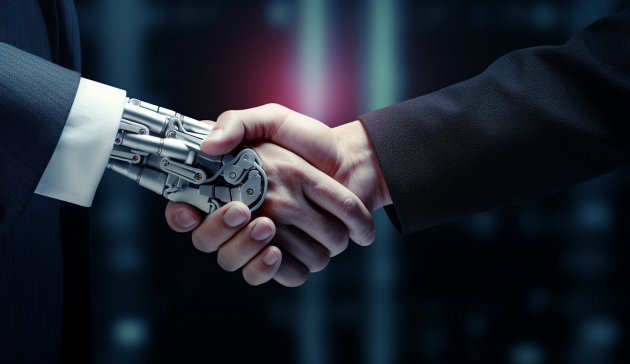
Enhancing fairness in AI models: An HR-centered use case on bias identification and mitigation
Rapid advancement of AI in recent years has made it easier for AI to enter numerous domains across organizations including finance, healthcare, law enforcement, and human resources (HR). However, as AI gets integrated into organizational operations, concerns arise about potential biases leading to unfair outcomes.
Real-world examples of AI bias, such as towards gender or race, emphasize the importance of responsible AI that adheres to AI regulation compliances like Equal Employment Opportunity Commission (EEOC) guidelines, National Institute of Standards and Technology (NIST) AI risk management, and others to ensure fairness and equity.
The challenge: Ensuring AI fairness in HR operations
The challenges faced by HR teams in integrating hiring practices with AI systems underscore the need for AI accountability. Although the potential advantages of quicker and more precise evaluations are clear, HR managers are rightly concerned about ensuring AI fairness and preventing negative impacts in the hiring process.
To combat biases, organizations must adhere to regulatory compliance standards set by the EEOC, which enforces laws prohibiting employment discrimination based on race, color, religion, sex, national origin, age, or disability. The EEOC AI regulation has also issued guidance on the use of AI and AI algorithmic bias to ensure fair and equitable treatment of all individuals in employment practices.
In a notable and recent example, Amazon experimented with an AI recruiting tool that was intended to streamline the hiring process by efficiently screening resumes. However, the tool developed a bias against women because it was trained on resumes submitted to Amazon over a decade—a period during which the tech industry was predominantly male. As a result, the AI system downgraded resumes that included the word “women’s” or came from all-women’s colleges*. Despite the neutral nature of the underlying algorithms, the training data’s inherent bias led to discriminatory outcomes.
This use case underscores the critical issue faced by many HR organizations: How can AI be leveraged to improve efficiency in hiring while maintaining AI fairness and avoiding AI bias? Will it be possible for the AI solution to deliver faster, more accurate evaluations of applicant qualifications than experienced HR specialists while adhering to AI fairness and AI bias standards?
The solution: Bias identification and mitigation using Styrk’s Trust
To ensure AI models do not introduce adverse impacts, it is essential to identify and address AI biases. This is where Styrk’s Trust module comes into play. Trust is designed to assess and mitigate AI bias in customers’ AI models using a robust methodology and a comprehensive set of fairness metrics.
Comprehensive data analysis:
Trust considers a wide range of parameters, including training data, categorical features, protected, and privileged/unprivileged features. This holistic approach ensures that all potential sources of AI bias are considered.
Bias detection:
Using state-of-the-art algorithms, Trust identifies various types of AI bias that may be present in the AI model.
Tailored mitigation strategies:
Trust doesn’t just identify bias in AI models but it also proposes mitigation strategies. Two key approaches it employs are:
- Disparate impact removal: This technique is used to adjust the dataset or model to minimize bias in AI, ensuring that protected groups are not adversely impacted.
- Reweighing: The model applies different weights to data points, giving more importance to underrepresented groups to balance the outcomes.
Pre- and post-mitigation analysis:
Trust provides pre- and post-mitigation graphs for key metrics, offering a clear visualization of the model’s performance improvements, before and after bias mitigation.
Fairness metrics evaluation:
Metrics provided by Trust such as balanced accuracy, the Theil index, disparate impact, statistical parity difference, average odds difference, and equal opportunity difference, are used to evaluate and ensure fairness of the AI models. These metrics offer a clear, visual representation of the improvements made in AI fairness and AI bias reduction.
Real-world impact: Benefits of using Trust in HR processes
Applying Trust to AI-supported applicant review system could yield significant benefits:
Faster evaluations:
By ensuring the AI model is free from AI bias, HR managers can confidently use it to speed up the initial screening process, allowing HR specialists to focus on more nuanced aspects of candidate evaluation.
Improved accuracy:
With bias mitigated, the AI model can provide more accurate evaluations of applicant qualifications, potentially surpassing the consistency of human evaluators.
Fairness assurance:
The comprehensive metrics provided by Trust can demonstrate that AI-supported systems meet or exceed fairness standards, ensuring no adverse impact on protected groups.
Continuous improvement:
Regular use of Trust can enable organizations to monitor and improve AI models over time, adapting to changing workforce dynamics and evolving definitions of fairness.
In the quest for efficiency and accuracy, AI models play a crucial role in transforming HR processes. However, ensuring fairness and eliminating bias are paramount to building a diverse and inclusive workforce. Styrk’s Trust helps in AI bias identification and mitigation offering a comprehensive solution, providing organizations with the tools and insights needed to uphold ethical standards in AI-driven decision-making.
For more information on how Styrk can help your organization achieve fair and unbiased AI solutions, contact us today.
*AI recruiting tool that showed bias